A CFO’s Guide to Setting up a Robo-Auditing System
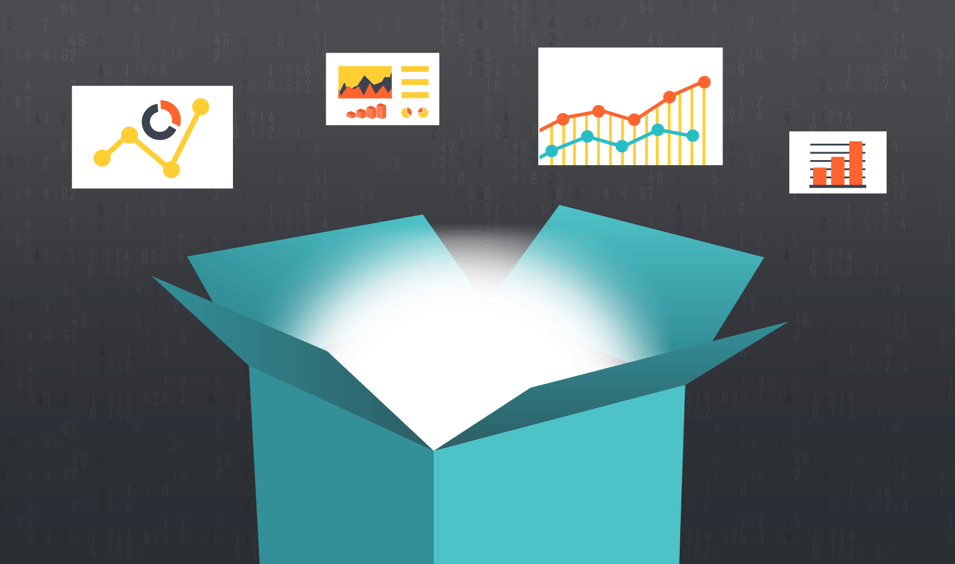
Artificial intelligence is making it possible for CFOs to improve their corporate financial systems in ways that once would have been unimaginable.
The mountains of data produced by corporate financial systems—transactions, potential problems, negative evidence—are a seemingly insurmountable burden for human auditors.
But robo-auditors powered by AI-and-automation systems can scale this mountain faster, more accurately, and for less cost than their human counterparts.
Furthermore, corporate finance is a perfect industry for robo-auditors because those years of structured, consistent financial data are just what a robo-auditor needs to be effective. It’s the equivalent of having a race car with plenty of fuel to make it go.
CFOs ready to implement a robo-auditing system typically have two big questions:
Here’s some guidance on how to set up a robo-auditing system at your company.
Do We Need to Design Our Own System?
For most companies, it makes more sense to purchase a packaged AI auditing system than to design their own. Most companies already have packaged general ledger applications, so why not a packaged robo-auditing system?
Problems caused by travel and entertainment expenses and accounts payable are common enough across industries that packaged robo-auditing systems make sense.
Even if your company employs data scientists, they are likely to be assigned to strategic projects. For example, a pharmaceutical company might assign a data scientist to a drug discovery project.
As CFO, you may not be able to get your data scientists to break away from their normal work to design an AI auditing system, maintain it, and improve it in the future. Plus, auditing probably can’t be their sole focus, and may not be one of their core competencies.
Those companies that build packaged systems are entirely focused on AI auditing. They learn from working directly with companies and fold that knowledge into their systems.
Prebuilt systems are easier to implement quickly, and the engineers who build them are continually improving the system. Packaged systems get better with time.
These continual improvements come from looking across multiple clients in different countries facing a variety of scenarios. Their data is far beyond what any individual company would have, and this makes their analysis more accurate and nuanced.
How Do We Put Our System to Work?
Another reason to use a packaged system is that it can immediately start working with a company’s rich data sets to set up accurate and reasonable safeguards that would be laborious for their teams to create and expensive to maintain.
For example, you can get in trouble for taking a government official out for a string of extravagant meals. So, your company has to ensure its employees entertain government officials in a way that is normal and customary for the locale.
But what constitutes a normal expense in these circumstances? What’s normal in New York City might be extravagant in Sioux Falls, South Dakota. For international travel, there’s exchange rates and inflation to consider, which varies by country.
These factors impact what is considered a reasonable amount to spend on dinner, and while you could manually code specific rules for every major city on the planet, it would be expensive and require ongoing effort to update the rules as factors changed.
However, with a rich data set, an AI system can use machine learning techniques to establish a reasonable amount for a meal in any given country or city.
The task is faster, doesn’t require nearly as much coding, and the information stays current because the computer keeps learning as it sees more expense transactions. The AI system recognizes trends in various global locations and the allowed reasonable amount for meals will change as the algorithms learn on an ongoing basis.
This process applies to hotel expenses as well, which are constantly in flux.
For example, it’s expensive to reserve a hotel in New York in December or July, but less so in February. Phoenix will be expensive in the winter but cheaper in the summer.
Computers are more efficient for learning and identifying reasonable hotel expenditures. The more data you collect, the better it gets. This is the network effect kicking in.
Whenever a robo-auditor flags an issue for human auditors to review, it’s an opportunity for the system to learn from the decision the auditor makes.
Remember that data is not useful unless it’s followed up with action.
This is why we recommend implementing your robo-auditing system in an area of your company that is straightforward, like travel and entertainment, before moving on to more complicated areas like accounts receivable. It gives your team an opportunity to learn how to use the system and provides some easy wins for the company to build on.
This is the sixth blog in an ongoing series based on the recently released book, Robo Auditing: Using Artificial Intelligence to Optimize Corporate Finance Processes by Patrick J.D. Taylor, Manish Singh and Nathanael L’Heureux.
The most sophisticated AI model in the finance and accounting space. Oversight AI supercharges your team's performance to control spend, ensure process integrity and compliance, eliminate fraud, waste, and errors, and do it all with less effort.