The 4 Types of AI That All CFOs Need to Know About
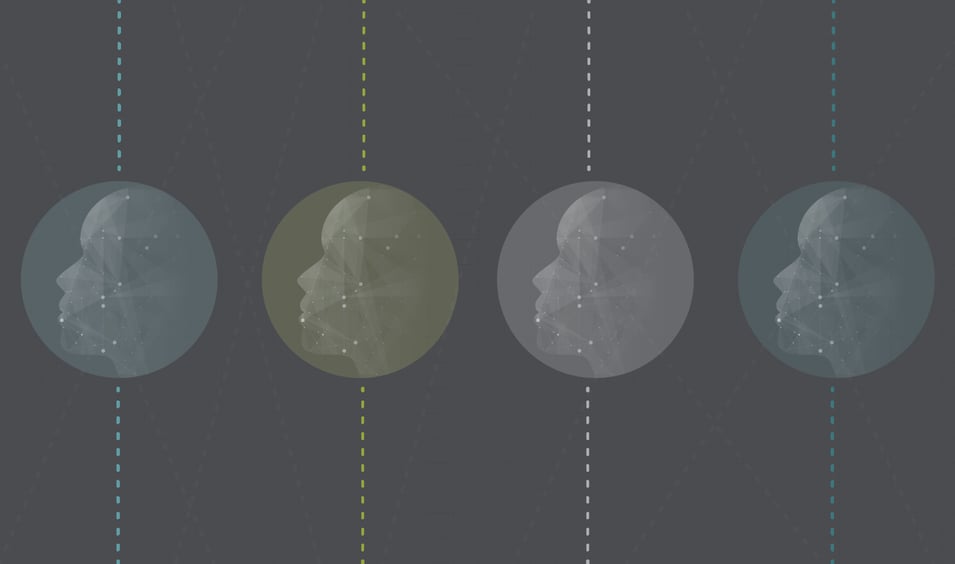
If you’re a CFO, you’re probably familiar with the concept of using a financial auditing system that incorporates artificial intelligence, automation, and robo-auditors.
The days of human auditors churning through data are slowly giving way to a future in which AI provides 24/7 monitoring of your company’s financial records to ensure that big issues (fraud) and small issues (incorrect expense reports) alike are flagged.
One of the biggest stumbling blocks to that better future is a lack of understanding.
For many of the CFOs we talk with, AI seems far too complex to implement within their company. The work these systems do is so comprehensive it seems like magic.
This article serves as a primer of sorts for CFOs who are intrigued by AI but need more familiarity with how it works before they’ll feel comfortable buying a system.
We’ll look at the four types of AI – expert system, evidential reasoning, neural networks, and machine learning – and see examples of how each one works.
Expert System: Diagnosing an issue
The most basic form of AI, an expert system codifies the rules a subject-matter expert uses to diagnose a problem. An expert, for example, might use a series of questions to narrow down his choices and reach a straightforward, yes-or-no answer.
It’s like a game of 20 Questions. Initial queries are often broad, but gradually narrow as the expert whittles away the possibilities to reach a reliable answer.
The challenge with this type of system is that specific rules take a long time to set up. Additionally, as things change, maintaining and adjusting the rules is laborious work.
The benefit of an expert system is that once the rules have been codified, they work tirelessly, accomplishing what an expert can accomplish faster and without error.
It’s still AI, but it’s so simplistic that most CFOs should look at the next three options if they’re serious about leveraging an AI system in their company.
Evidential Reasoning: Detecting problems
A system using evidential reasoning is more dynamic than an expert system because it’s designed to find problems in gray areas, not just using black-and-white rules.
Evidential reasoning operates like a detective, looking for clues and applying weights to each hint to make some pieces of evidence more significant than others.
Clues are known risk factors, both quantitative and qualitative, within the data. A quantitative clue is measured numerically, such as when one salesperson upgrades to a luxury car at three times the normal rate of other employees.
A qualitative clue requires judgment, such as when you’re asked to change a vendor’s account to a Caribbean bank. That’s not necessarily wrong, but it is worth checking.
The system looks for these types of risks, and the more risk factors it discovers, the higher your confidence is that the system has found something legitimately wrong.
For example, many companies have a rule that states employees don’t need a receipt for any expense under $25 dollars. Your system can look for employees who are cheating and making up expenses that don’t require a receipt.
One clue it might find is that one employee has three times more sub-$25 expenses than other employees. If every breakfast, lunch, and taxi ride is uncommonly close to $25, you can have increased confidence that this person is up to something.
You can also find problems with vendor invoices. For example, two large corporations in the technology space were reportedly defrauded of $100 million over a two-year period by a Lithuanian man posing as a legitimate vendor.
To catch a situation like this, a robo-auditor would identify clues such as a changed bank account or mailing address. It would search for an abnormal email address that didn’t match up with that vendor’s previous data, or it could have looked for a vendor invoice number that was out of order or had a different pattern than the vendor’s normal invoices.
The term “clues” suggests that you’re always looking for wrongdoing, but you can also use these indicators to find new sales opportunities.
For example, when a customer opts in on your website to download a white paper, then signs up to attend your webinar, the AI system will assign them a heavier weight than someone who only visited your website and downloaded the white paper.
Neural Networks: Tracking patterns and sequences
If you’ve ever gotten a call from your credit card company to see if recent transactions on your account were fraudulent, you’ve experienced the power of neural networks. Credit card companies are pioneers in using large data sets to prevent fraud.
For example, if your card is swiped at the gas station and liquor store before being used to purchase 18 big screen TVs from the electronics store, you’re getting a call.
After years of tracking the patterns of fraudulent purchases on stolen credit cards, the AI system used by card companies know to flag that sequence of transactions.
Neural networks like this recognize patterns and mimic how the brain works.
You start by feeding a big set of training data into the network (e.g. decades of credit card purchases by millions of customers) and identifying the important outcomes.
The neural network then develops its own logic for how to predict those outcomes based on the input data. The more data you feed it, the more accurate its results.
You may go through several iterations of training a neural network on data sets before you turn it loose on real-world data, like monitoring credit card purchases.
Machine Learning: Identifying patterns
Machine learning is when a machine is programmed to learn in the same way humans learn and makes course corrections based on the data it collects.
For example, say you drive three different routes to work, each one different:
After driving these three routes for a while, you take a step back and weigh the positive and negative characteristics of each one. As humans, we do this all the time.
The same pattern can work in a machine. In the first analysis, it looks for the anomalies that don’t fit in the parameters we have set up.
In accounting, for instance, the machine is looking for risks in financial transactions, based on an initial set of rules and known patterns of risk. At this first level, the machine might flag a thousand transactions that might have some risk.
Then the machine moves on to that second level. It watches how humans treat each of those thousand transactions. It sees that some are deemed safe while others are escalated as serious problems. The machine learns what are real problems and what aren’t, and it automatically adjusts the criteria it uses to flag a potential problem.
In deep machine learning, there is even a third level where the machine uses the knowledge it has gained and refines the underlying rules on the first level. Lessons learned are fed back in and affect the first order of analysis.
Supervised learning is when a human is running data sets through the machine and teaching it what’s right and what’s wrong. Unsupervised learning doesn’t require that complex pre-training data. Instead, the machine is looking at real-life data, suggesting outcomes, and learning as a human steps in and says yes or no to what the machine has suggested. The machine is constantly learning and refining its outcomes.
A robust robo-auditing system, like Oversight’s Insights On Demand, is already very smart right out of the box. It gives good results immediately, because we know enough about the domains they work in—such as travel, expense, purchase card, or accounts payable—to program in a strong set of first-level analytics. There are well-known best practices for these domains.
But even that well-trained, out-of-the-box system will keep improving and learning as it watches how you process the situations it surfaces. It’s always learning and never gets tired. Read on to find out how this combination of artificial intelligence and automation can help you get more horsepower out of your company’s engine.
This is the third blog in an ongoing series based on the recently released book, Robo Auditing: Using Artificial Intelligence to Optimize Corporate Finance Processes by Patrick J.D. Taylor, Manish Singh and Nathanael L’Heureux.
Chief Solutions Ambassador